Cecilia Rosso
- Dottore in Scienze Farmaceutiche e Biomolecolari
- Phd: 23rd cycle
- Matriculation number: 337322
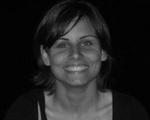
Contacts
- +39116704645
- cecilia.rosso@unito.it
- Via Accademia Albertina 13
- https://dott-sfb.campusnet.unito.it/do/studenti.pl/Show?337322
- VCard contacts
- QRcode contacts
Supervisor
Francesca ValettiPhd thesis
Definition of in silico predictive methods for xenobiotics recognition properties of engineered mutants of a non-heme iron dioxygenase. The in silico prediction of enzyme activity and selectivity is a complex issue since it involves both the identification of the structural motifs in substrates and enzyme that are discriminative and the computation of suitable quantitative descriptors of structure/activity relationship. Most promising results should arise from the joint application of chemometrics –i.e. multivariate analysis or QSARs - and molecular simulation methods for the in silico calculation, in synergy with an experimental model suited for testing the predictive model. The proposed test case, a non-heme iron enzyme of which at least eight different active site mutants are available with varied substrate selectivity on chlorinated aromatics, fits even further the complex systems research field adding the possibility to explore the potentialities of computational methods to design novel and optimised biocatalysts. The aim of the project is to define an in silico predictive strategy of enzyme selectivity for biocatalysis using as input data the experimental characterisation of active site designed mutants of catechol 1,2 dioxygenase Iso B (C1,2O) from the bacterium A. radioresistens S-13. Catechol 1,2 dioxygenase (C1,2O) is a non-heme iron dioxygenase able to catalyse the ortho cleavage of the diolic aromatic ring leading to a non-toxic metabolite and it represent a potential biocatalysts of great interest in degrading toxic compounds (pollutants, drugs and other xenobiotics). Protein-engineering was successfully applied to increase the range of substrates recognised by C1,2O. A 3D model of the active site of C1,2O was generated by homology modelling and 8 active mutants were obtained. The activity of the purified mutants was assayed to determinate the kinetic constants for the natural substrate, methylcatechols, chlorocatechols and halogenated catechols Steady-state kinetic studies demonstrated that the target residues are crucial to various extent both for substrate recognition and the various aminoacid substitutions differently affect Km and kcat. Most of the efforts of computational studies in the field of biocatalysis aims at the prediction of enzyme selectivity constants (kcat/Km), which depends on the free energy of the transition state of the reaction. The experimental values of the selectivity constants (kcat/Km) are available for both the wild type and the mutants of all cited substrates and will be used as input values for the in silico prediction strategy testing. The computation of free energy of the transition states in complex biocatalytic system is usually dealt with by a combination of molecular mechanics (MM) and quanto mechanics (QM) ab-initio methods. The latter are the most accurate but they can simulate only the behaviour of few tens of atoms. In proteins MM methods based on empirical force fields such as AMBER are more feasible for such computational simulation. A general high-throughput method for in silico biochemical profiling of enzymes is based on t docking of potential substrates into the binding sites. The AUTODOCK 4.0 programm will be applied which is based on AMBER, but encompasses also a Montecarlo simulated annealing and protein side chains flexibility. The description of the chemical nature of the active site in terms of binding capabilities will also be accomplished with GRID. Classical quantitative structure-activity relationships (QSARs) will be used to correlate the kinetic constants with molecular descriptors and the resulting model will be assessed for accordance with experimental data variability. A refinement cycle can be proposed based on the design of a further set of mutants whose substrate binding capabilities and selectivity constants, predicted by the in silico methods, will be experimentally tested and used as novel input data for computational method improvement.